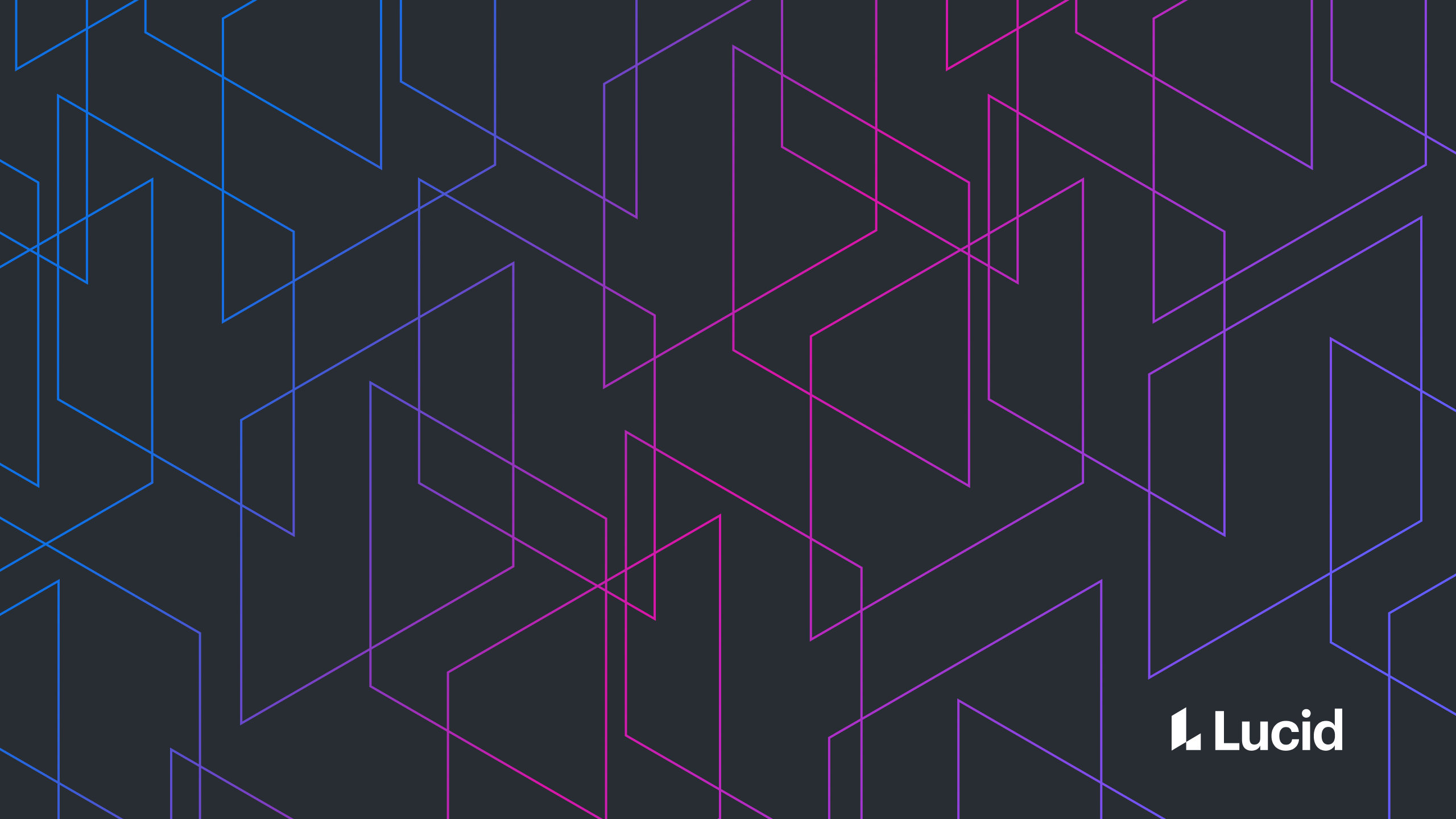
Using Analytics to Impact Product Decisions at Lucid
Remy Millman
Reading time: about 8 min
“Product analytics” can mean a lot of different things to different people. Intensive SQL modeling? Managing and analyzing user data? Running AB tests? Contributing to the vision of the product through strategic analysis? All of this and more can fall under the broad category of product analytics. Figuring out where all of these pieces fit into the bigger picture is a fun puzzle to solve.
That’s where my team comes in. My name is Remy Millman, and I lead our product analytics team here at Lucid. We are a part of the broader Strategy & Analytics team, specializing in helping out the product organization. Which brings up a question that may be on your mind...
What does an analytics team do?
The goal of the Strategy & Analytics team at Lucid is to drive impact through data analysis. “Impact” is a broad term we use for work that falls into at least one of the following categories:
- Move a metric
- Change a product
- Improve a process
If a project or initiative involves data and falls into one of those categories, then it’s our job! Whenever we spend time on a project or analysis, we focus on its impact for the company. This helps us focus our attention on what matters most to the organization.
On the analytics team, we take a hypothesis-based approach to our projects in order to ensure that our analysis helps drive the intended impact. This means that we plan out our analysis at the beginning in support of defined and scoped assertions that support our overarching hypothesis. We have so much data available at Lucid and an endless number of ways to slice it, so this upfront planning is essential to making sure that we produce valuable output. Framing our analysis and asking the right questions is a huge part of what makes any analytics project successful.
How does the product team approach decision-making?
Before we can actually help the product team make decisions using analytics we need to understand how they make decisions in the first place. The role of a product manager is to set the vision for the product, and this involves making a ton of decisions about a variety of topics. At the core of this, PMs are trying to identify problems that our users are trying to solve and how our products can help.
An important part of this process is developing and testing hypotheses. A hypothesis in this context often takes the form of “We should implement X” or “We should devote resources to improving Y.” Product managers will come up with these hypotheses based on user research or intuition, and then they can test them.
The testing of the hypotheses is where the data comes in! This can take on many forms, from running quick analyses, to conducting AB tests in the product, to doing deep-dive analytics projects.
Quick analyses happen all the time. One example would be a funnel of steps in a user flow. Our hypothesis may be that users are falling out of the funnel at a particular step, so we can look in the data to validate this hypothesis. This can be a powerful starting point for product decisions, as this helps inform us where in the user flow the product needs to be improved.
Running AB tests is another common form of using data to inform product decisions. An AB test takes a group of users and randomly assigns them to different experiences in the product. Then we observe key metrics to see the difference between the groups of users. AB test analysis is a more statistically rigorous way to attribute causation to results seen from product changes. For example, let’s say we want to change the design of the Lucid home page to a different color because we believe it will increase engagement with our products. We could run an AB test, simulating a scientific experiment and test the effect this has on overall product engagement. If engagement goes up, we can conclude that this uptick was the result of our product changes, which would validate our initial hypothesis. This enables the product team to make an informed decision on how to proceed with this change, knowing that it has a positive effect on engagement based on empirical results.
Another way in which product analytics helps with decision-making is in deep-dive analytics projects. These projects come about when there are ambiguous problems to be solved and decisions to be made. In these cases, the analytics team will work with product managers to frame the problem and break it down into hypotheses and assertions that can be analyzed with data. One example of a project like this was a few years ago when product leaders wanted to know what we should be doing from a product standpoint to ensure that our largest enterprise accounts can effectively grow. This triggered a landmark Lucid analytics project called “How Accounts Grow” which tackled several hypotheses related to this question. The end result of this was a handful of actionable recommendations about how to allocate resources on the product team. Years later, it’s been clear that this analysis was extremely influential in shaping the roadmap and eventually changing the product itself to support account growth.
What does a product analytics project look like in action?
One recent example of a successful product analytics project was an analysis on using Lucidspark as a Team Hub, led by one of our amazing analytics interns this summer. The background behind the project was that there had been some qualitative research done indicating that people were trying to use our product as a “hub” for their team. The purpose of the analytics project was to investigate how frequently this was happening and to come up with recommendations for how to improve Lucidspark to facilitate this use case.
The first step of the process was to work with product stakeholders to understand the different flows that users take to make their Lucidspark boards into team hubs. This enabled us to define the team hub flows in our data.
Once we had our definitions down, we developed quantifiable hypotheses and assertions to plan out our analysis. These assertions focused primarily on which types of features and usage patterns led to success in the team hub use case. Our primary hypothesis was that we should be focusing more on implementing features that help users bring outside content into Lucidspark, such as embedding functionality. We even went so far as to mock up what our final slide deck would look like in order to streamline the data analysis portion of the project. These “blank slides'' outlined what exact data and visualizations we would need to tell our story. This process helps to frontload the data planning and reduce the chances of going down analytical rabbit holes.
After we had established what analysis we were planning on doing for the project, it was time to dig into the data! This involved writing up SQL queries according to our work plan and creating visualizations in Tableau. Once we had done all of the analysis, we crafted a final report with our findings and recommendations to tell the story of how certain types of features impacted the success of users using Lucidspark as a team hub.
The project concluded with a discussion between the stakeholders on the product team. We discussed our findings from the analysis and how this would impact the future of the product roadmap. At the end of the meeting, the product managers were already chatting about how they should alter their plans for the following quarter to focus more on our recommendations around prioritizing embedding functionality in Lucidspark. This type of decision-informing impact is exactly what makes a product analytics project successful!
What does the future of product analytics look like at Lucid?
It’s a super exciting time to be working with the product team at Lucid! The organization continues to grow as we build out our visual collaboration suite, and the product analytics team is growing right along with it. In the past five months, we’ve added four new fantastic full-time analysts who are already contributing to impactful projects.
As we grow the team, we will continue to focus on thought partnership with the product managers. Our goal is to help inform the biggest product decisions using data, so this partnership is essential to making sure we are moving in the right direction. This means knowing the priorities of the product org and forming the necessary relationships to increase the trust between analysts and PMs.
Another important aspect of the future of product analytics is trust and transparency in the data itself, as well as accessibility of the data for our stakeholders. We will continue to invest heavily in our internal event-tracking system to ensure that data is well-planned and reliable. Additionally, we will expand on the tools that people use to easily access and analyze this data. The ability to self-serve for quick analytics questions is a huge part of what makes the relationship between product and analytics successful. When PMs can answer their own questions, it enables the analytics team to focus on the more big-picture questions and projects.
At the end of the day, the product analytics team’s effectiveness is a function of the quality of our analysts and the trust the product org has in them. As the company scales and our product offerings become even more robust, it is integral that our data and analytics processes scale with it. It’s an exciting time to be in product analytics at Lucid, and I couldn’t be more excited for the future of this team!
About Lucid
Lucid Software is a pioneer and leader in visual collaboration dedicated to helping teams build the future. With its products—Lucidchart, Lucidspark, and Lucidscale—teams are supported from ideation to execution and are empowered to align around a shared vision, clarify complexity, and collaborate visually, no matter where they are. Lucid is proud to serve top businesses around the world, including customers such as Google, GE, and NBC Universal, and 99% of the Fortune 500. Lucid partners with industry leaders, including Google, Atlassian, and Microsoft. Since its founding, Lucid has received numerous awards for its products, business, and workplace culture. For more information, visit lucid.co.